AI and plant science: Transforming greenhouses
Added on 24 October 2024
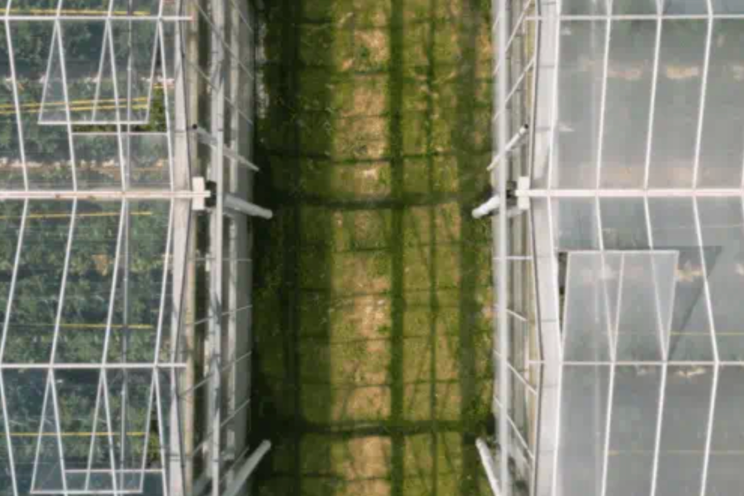
The role of algorithms in modern greenhouses
One of the most innovative uses of AI in greenhouse cultivation today involves algorithms that analyze vast amounts of plant and environmental data to generate actionable insights. Source.ag is among the few companies at the forefront of this approach, applying AI-driven models to optimize key factors in greenhouse management.
In high-tech greenhouses, AI algorithms excel at monitoring and optimizing variables such as water usage, temperature, and humidity—factors critical for maintaining plant health and maximizing yield. By leveraging data from environmental sensors, these AI models can predict plant behavior and make real-time adjustments that ensure optimal growing conditions.
A prime example of this is the use of AI for irrigation management. By collecting and analyzing data on water uptake, radiation, humidity, and other environmental factors, AI models continuously predict the exact water needs of each plant. This data-driven approach enables greenhouse operators to refine irrigation strategies in real time, making sure that plants receive precisely the amount of water they need at every stage of growth. By doing so, the AI not only conserves water but also helps prevent over-irrigation, which could cause problems such as root disease and reduced crop quality.
Predicting yield with artificial intelligence
Yield forecasting is another area where significant advancements with AI are being made by a few leaders in the market, including Source.ag. Accurate yield predictions are essential for both operational planning and financial forecasting, yet traditional methods relying on historical data or manual estimates often lead to inaccuracies.
More news